You’ve decided to tackle “data governance” in 2024. Where do you start? What are some things the organization needs to think about to create a wholistic governance strategy?
Let's delve into a detailed discussion about the stakeholders impacted by data governance, identify the key factors that need to be considered for effective data governance, and outline a step-by-step approach for implementing a comprehensive data governance strategy.
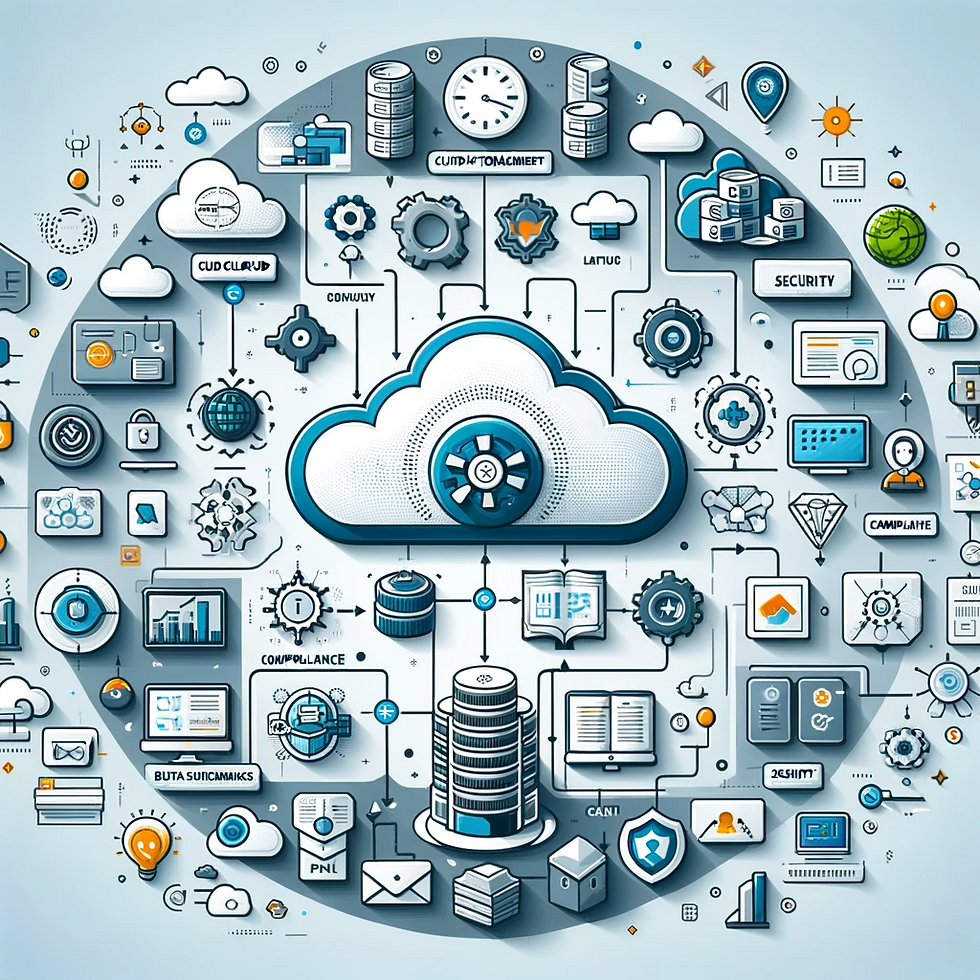
Get To Know Your Organization’s Users
The following illustrates various user profiles and their specific data requirements. It's crucial to recognize how data quality and security impact a wide range of individuals in their daily tasks. A fundamentally flawed data governance framework can lead to inconsistencies in reporting and the reliance on inaccurate data for critical decision-making processes. Understanding these dynamics is key to ensuring that data-driven strategies are both reliable and effective.
Business User | Data Analyst | Data Engineer | Data Scientist |
Self-service analytics | Conducting demand analysis | Implementing real-time data processing capabilities | Experimenting with new models and techniques |
Customer 360 views | Managing self-service data pipelines | Focusing on data quality and lineage | Evaluating different data models for best fit |
Personalization strategies | Integrating machine learning capabilities with SQL | Choosing the right tools and languages for different tasks | Making predictions based on data analysis |
Customer Satisfaction Analysis (CSAT) | Performing analyses across diverse data types | Developing self-driving data infrastructure for automation and efficiency | Retraining models to stay relevant and accurate |
The Three Major Issues Around Data Governance
There are several challenges that impede effective data management. One of the primary issues is the presence of 'dark data' - information that is collected but remains unused. This often includes data from outdated projects or data that hasn't been properly indexed, rendering it difficult to locate and use efficiently.
Another critical aspect is the quality and completeness of data. Bad or missing data can significantly skew analyses, leading to erroneous business decisions. Therefore, ensuring data accuracy and completeness is a fundamental aspect of data governance.
Moreover, the consistency in applying data governance policies plays a pivotal role in maintaining data integrity. Inconsistencies in policy application can lead to data discrepancies and unreliability, significantly impacting the data's trustworthiness and usability. Addressing these challenges is crucial for organizations to leverage their data assets effectively and make informed decisions.
Processes for Effective Data Governance
Effective data governance in the AI era involves a series of critical processes. It starts with the automatic discovery and classification of data and its metadata, which lays the foundation for effective governance. Organizing this data into specific domains not only streamlines management but also enhances its utility. To further increase the data's relevance and value, it is enriched with business context, making it more applicable for business decisions. Trust in the data is established by ensuring its quality and understanding its lineage, aspects vital for reliable data usage.
Data curation is tailored to align with specific organization policies and needs, ensuring a personalized and effective governance strategy. Protecting this data is crucial, achieved through dynamic, metadata-driven policies. Additionally, continuous monitoring and auditing of data are essential to maintain its integrity and relevance, ensuring accuracy and up-to-dateness.
Data now comes in multiple formats and vast quantities, requiring robust governance strategies that can handle such diversity. This governance goes beyond SQL databases, dealing with complex data types and structures. The quality of data affects every member of an organization, making high data quality crucial for overall business health.
High-quality data is essential for unbiased AI models, leading to more accurate and reliable outcomes. AI models should be based on high-quality data rather than focusing solely on training data to ensure they are built on a solid foundation. This approach to data governance ensures that data-driven decisions are both insightful and trustworthy, propelling businesses forward in the AI-driven world.
Sample Implementation Plan for GCP or Azure
Step 1: Automatic Discovery and Classification of Data
Objective: To lay the foundation for data governance by automatically identifying and classifying data and metadata.
Stakeholders: Data Engineers, IT Security Team.
Technologies: Azure Data Catalog for data discovery, Azure Purview for automated classification and governance in Azure. Google Cloud's Data Catalog and Cloud Data Loss Prevention (DLP) for similar functionalities in Google Cloud.
Action Items:
Deploy Azure Data Catalog or Google Cloud's Data Catalog to discover data assets.
Utilize Azure Purview or Cloud DLP to classify data and metadata.
Step 2: Organizing Data into Specific Domains
Objective: Streamline data management and enhance its utility by organizing it into specific domains.
Stakeholders: Data Architects, Business Analysts.
Technologies: Azure Blob Storage, Google Cloud Storage for data organization; Azure SQL Database, Google Cloud Bigtable for structured data management.
Action Items:
Define data domains based on business needs.
Organize data into these domains using the chosen storage solutions.
Step 3: Enriching Data with Business Context
Objective: Increase data's relevance and value by adding business context.
Stakeholders: Business Intelligence Teams, Data Analysts.
Technologies: Azure Synapse Analytics, Google Cloud's BigQuery for data warehousing and enrichment.
Action Items:
Integrate business context into data sets using data warehousing tools.
Collaborate with business units to ensure relevant enrichment.
Step 4: Ensuring Data Quality and Understanding Lineage
Objective: Establish trust in data by ensuring its quality and understanding its lineage.
Stakeholders: Data Stewards, Compliance Officers.
Technologies: Azure Data Factory, Google Cloud Dataflow for data lineage; Azure Data Quality Services, Google Cloud Data Quality for data quality
management.
Action Items:
Implement data quality tools to clean, monitor, and manage data quality.
Use data lineage tools to track the origin and transformation of data.
Step 5: Tailoring Data Curation to Organization Policies
Objective: Personalize data governance to align with specific organizational policies and needs.
Stakeholders: Policy Makers, IT Administrators.
Technologies: Azure Policy, Google Cloud's Policy Intelligence.
Action Items:
Develop and enforce data governance policies that reflect organizational requirements.
Leverage policy management tools to ensure compliance and alignment.
Step 6: Securing Data with Metadata-Driven Policies
Objective: Protect data dynamically through the use of metadata-driven policies.
Stakeholders: Security Teams, Data Managers.
Technologies: Azure Security Center, Google Cloud Security Command Center.
Action Items:
Implement security policies based on data classification and metadata.
Regularly review and update security policies to adapt to evolving threats.
Step 7: Continuous Monitoring and Auditing of Data
Objective: Maintain the integrity and relevance of data through continuous monitoring and auditing.
Stakeholders: Audit Teams, IT Operations.
Technologies: Azure Monitor, Google Cloud Operations Suite.
Action Items:
Set up continuous monitoring systems for real-time data tracking.
Conduct regular audits to ensure data integrity and compliance.
Step 8: Handling Diverse Data Formats and Volumes
Objective: Manage the diversity and volume of data in various formats.
Stakeholders: Database Administrators, Data Integration Teams.
Technologies: Azure Cosmos DB, Google Cloud Firestore for handling diverse data formats; Azure Databricks, Google Cloud Dataproc for big data processing.
Action Items:
Implement database solutions that can handle various data formats.
Use big data processing tools to manage large volumes of data.
Step 9: Establishing High-Quality Data for AI Models
Objective: Ensure the development of unbiased and accurate AI models based on high-quality data.
Stakeholders: Data Scientists, AI Model Developers.
Technologies: Azure Machine Learning, Google Cloud AI Platform.
Action Items:
Develop AI models using platforms that prioritize high-quality data input.
Regularly evaluate and retrain AI models to maintain accuracy and relevance.
Reference:
Comments