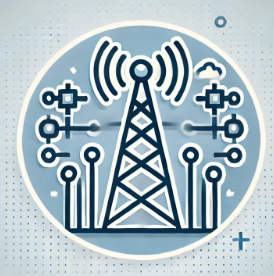
Artificial Intelligence (AI) is transforming industries, and telecom is no exception. From optimizing network performance to predicting outages and improving customer experience, AI has the potential to revolutionize how telecom companies operate. But there’s one big question every telecom executive faces: Should we build our own AI tools, or should we buy readymade software? It’s not a one-size-fits-all answer, and understanding the pros and cons can help you make the best decision for your business.
A recent study by Hoffreumon, Forman, and van Zeebroeck (2024) dives deep into this very issue, analyzing how firms decide between building AI solutions in-house, buying them off the shelf, or doing a bit of both. Using data from over 3,000 firms across Europe, the authors examine what works for different industries and why. Let’s break it down into digestible chunks so you can see how this might apply to the telecom industry.
The Case for Buying AI Software
Let’s start with buying. Readymade AI software is exactly what it sounds like—tools that are pre-built, standardized, and ready to deploy. Think of it like buying a suit off the rack. Sure, it might not fit perfectly, but it’s quick, easy, and usually much cheaper than custom tailoring.
The study found that 58.3% of firms adopting AI reported using readymade solutions, which are popular for good reason. These tools come with built-in best practices and are often designed by companies that have years of experience solving similar problems for a variety of clients. "By accumulating best practices from multiple engagements, readymade software may also help facilitate the business process innovation that commonly accompanies enterprise software adoption" (Hoffreumon et al., 2024, p. 453).
For telecom companies, readymade software can address challenges like network optimization, customer churn prediction, and service automation. These solutions are often backed by vendors who specialize in telecom use cases, making it easier to deploy them quickly and see immediate benefits. For example, many off-the-shelf AI tools for telecom are pre-trained to analyze call data records or optimize 5G deployment strategies.
That said, readymade tools have their limitations. They’re designed to be generic and may not align perfectly with your specific network architecture or operational processes. If your telecom company has unique needs, such as proprietary network protocols or a focus on niche markets, this could be a problem. Think of it like trying to fit a square peg into a round hole. Sometimes, a bit of customization is required to make the tool work for you.
The Case for Building AI Software
On the flip side, building AI in-house is like creating a bespoke suit. It’s tailored to your exact needs, but it takes time, money, and skilled labor to get it just right. According to the study, in-house development is more common in high-tech industries like IT and finance, and it’s increasingly becoming a trend in telecom as companies seek to differentiate themselves in a competitive market.
"Building software is expensive, and once developed, it is hard to change," the authors explain (Hoffreumon et al., 2024, p. 454). But for telecom firms, the benefits of building AI can be significant. Custom AI solutions can address complex use cases such as dynamic spectrum allocation, real-time fraud detection, and personalized customer recommendations. In a world where network reliability and user experience are paramount, having AI that’s perfectly aligned with your operations can be a game-changer.
However, building AI isn’t for everyone. You need skilled talent, which can be expensive and hard to find. The scarcity of AI expertise is a common hurdle, with the authors noting that "adoption will be constrained by a scarcity of talent or require costly training by the adopting firm" (Hoffreumon et al., 2024, p. 453). Additionally, the process takes time, so it’s not ideal for companies that need a quick solution.
Why Not Both? The Power of Complementarity
Here’s where things get interesting. The study reveals that building and buying aren’t mutually exclusive. In fact, many businesses use a hybrid approach, combining readymade solutions with in-house development. This strategy is particularly common in industries like scientific research, retail, and manufacturing—and it’s gaining traction in telecom.
The idea is that these two strategies can complement each other. For example, a telecom company might purchase a readymade AI tool for basic functions like network monitoring but develop custom features in-house to optimize specific network configurations or handle region-specific customer behaviors. "Complementarities between building and buying software may arise because in-house knowledge assets help firms better utilize innovations acquired elsewhere," the authors note (Hoffreumon et al., 2024, p. 455).
This hybrid approach allows telecom firms to leverage the cost-effectiveness of readymade solutions while still customizing critical components. For instance, many telecom companies use off-the-shelf AI for call center automation but build their own predictive maintenance models to reduce outages.
Sector-Specific Insights for Telecom
The telecom industry has unique challenges that influence the build-versus-buy decision:
Network Optimization: Readymade AI tools excel at tasks like traffic analysis and load balancing, but custom solutions might be needed for optimizing legacy systems or proprietary technologies.
Customer Experience: Buying off-the-shelf chatbots or sentiment analysis tools can address immediate needs, while in-house solutions might be required for deeper personalization.
Fraud Detection: Telecom companies often build their own AI for fraud detection to ensure it aligns with specific regulatory requirements and network protocols.
5G Deployment: AI-driven planning and simulation tools are often purchased, but custom-built solutions may be necessary for companies looking to differentiate their 5G services.
The authors also emphasize the importance of proximity to AI research institutions. Telecom firms located near leading AI universities or research centers are more likely to develop AI in-house because they have better access to talent and cutting-edge knowledge.
The decision to build or buy AI in the telecom industry isn’t just about software—it’s about strategy. As Hoffreumon and his colleagues point out, "AI adoption requires both technical expertise and business process innovation" (Hoffreumon et al., 2024, p. 455). Whether you choose to build, buy, or combine the two, the key is to align your AI strategy with your business goals and resources.
AI is a powerful tool for telecom companies, enabling better network management, improved customer experience, and faster innovation. By carefully evaluating your options, you can ensure that your AI investments deliver maximum value. So, what’s it going to be? Build, buy, or both?
References:
Hoffreumon, A., Forman, C., & van Zeebroeck, N. (2024). Make or buy your artificial intelligence? Complementarities in technology sourcing. Economics of Innovation and New Technology, 33(1), 452-465.
Comments